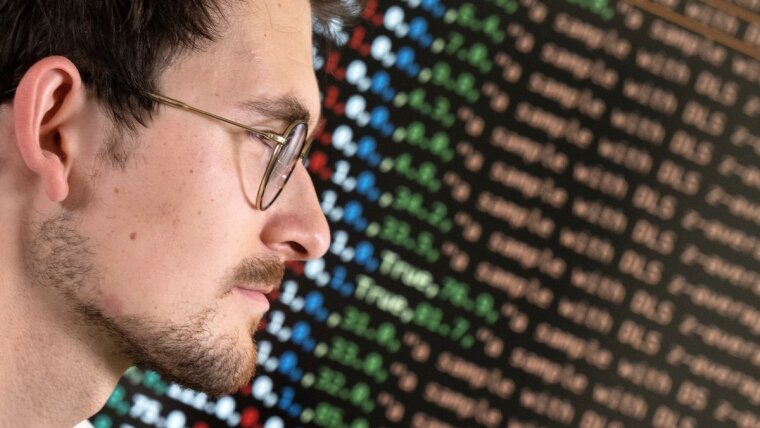
Published: | By: Marco Körner
Source article
GPT-3, the language model behind the well-known AI system ChatGPT, can also be utilised in chemistry to solve various scientific tasks. This was demonstrated by a team of researchers at the École Polytechnique Fédérale de Lausanne (EPFL), Friedrich Schiller University Jena, and the Helmholtz Institute for Polymers in Energy Applications (HIPOLE) Jena. As reported in the journal “Nature Machine Intelligence”, they circumvented the issue that chemistry often lacks the large datasets required for training an AI.
Curated Questions and Answers Instead of Large Datasets
“One of the various examples we used are so-called photosensitive switches,” illustrates Kevin Jablonka, lead author of the study. “These are molecules that change their structure when exposed to light of a certain wavelength. This type of molecule also exists in the human body: In our retinal cells is the molecule rhodopsin, which reacts to light and thus ultimately acts as a chemical switch converting optical signals into nerve impulses,” he adds. “Therefore, the question of whether and how an as yet unknown molecule can be switched by light is indeed relevant – for instance, when it comes to developing sensors,” he summarises. “We also addressed the question of whether a molecule can be dissolved in water,” Jablonka mentions as another example, “as water solubility is an important factor for pharmaceutical agents to exert their desired effect in the body.”
To train their GPT model to answer these and other questions, the group had to solve a fundamental problem: “GPT-3 is not familiar with most of the chemical literature,” Jablonka explains. “Thus, the answers we get from this model are usually limited to what can be found in Wikipedia.”
Instead, Jablonka continues, the group specifically improved GPT-3 with a dataset of relatively few questions and answers. “We thus fed the model with questions – for example, about photosensitive switchable molecules, but also regarding the solubility of certain molecules in water and other chemical aspects – where we also provided the respective known answer for our ‘teaching examples’,” he elaborates. In this way, he and his team created a language model capable of providing correct insights into various chemical issues.
Fast, Accurate, and Easy to Use
Subsequently, the model was tested. “The scientific question about a light-switchable molecule could look like this,” Jablonka clarifies: “What is the pi–pi* transition wavelength of CN1C(/N=N/ C2=CC=CC=C2)=C(C)C=C1C?” Since the model is text-based, structural formulas cannot be specified, he explains. “But our GPT works well with the so-called SMILES codes for molecules, as in the example above,” he says. “It also recognises other notations, including chemical names that follow the so-called IUPAC nomenclature, as one might remember from chemistry class,” Jablonka continues.
In tests, the model solved various chemical problems, often outperforming similar models that have been developed in the scientific community and trained with large datasets. “However, the crucial point is that our GPT is as easy to use as a literature search, which works for many chemical issues – such as properties like solubility, but also thermodynamic and photochemical properties like solution enthalpy or interaction with light – and, of course, chemical reactivity,” adds Prof. Dr Berend Smit from EPFL Lausanne.
Original publication:
Kevin Maik Jablonka, Philippe Schwaller, Andres Ortega-Guerrero, Berend Smit: "Leveraging large language models for predictive chemistry”, Nature Machine Intelligence 2023, DOI: 10.1038/s42256-023-00788-1External link